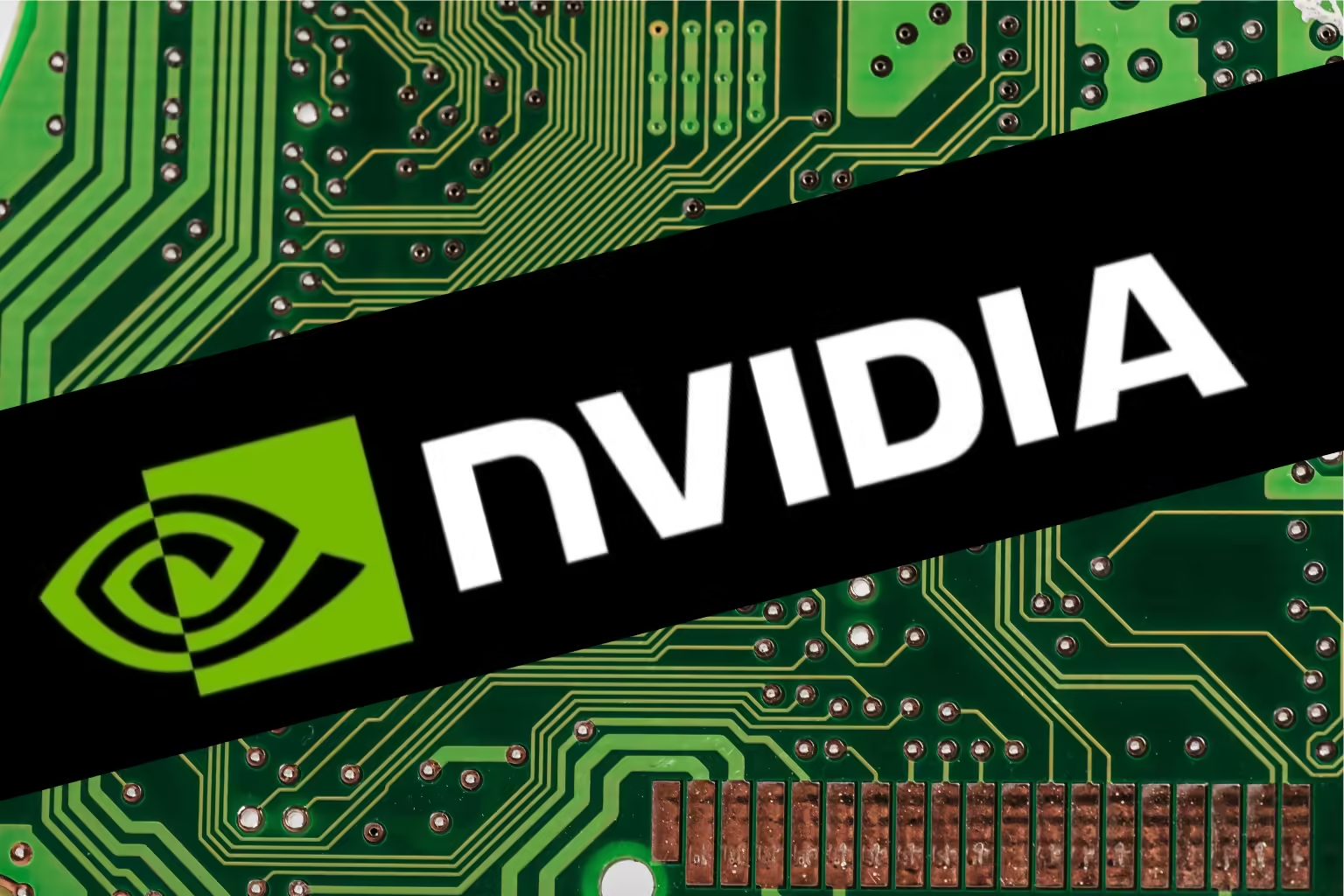
NVIDIA Unveils MM-Embed: Groundbreaking Multimodal AI Sets New Benchmark
The MM-Embed from NVIDIA sets a new standard for AI-powered multimodal retrieval by getting the best scores on the M-BEIR benchmark.
With the release of MM-Embed, NVIDIA is pushing the limits of AI in information retrieval. It is the first multimodal AI retriever to get state-of-the-art (SOTA) scores on the M-BEIR benchmark. Researchers at NVIDIA came up with this ground-breaking model that works great with a wide range of search queries that include both text and images. It opens up a whole new era of multimodal search powers.
Most traditional retrieval models can only work with one type of data, like text-to-text or image-to-image. Because of these limits, it’s been hard to use these apps in places where material needs to be correctly processed across formats, like when answering visual questions or finding fashion images. NVIDIA gets around these problems with MM-Embed, which makes cross-modal understanding seamless. This lets users do complicated queries that include both text and images.
A bi-encoder design and a method known as “modality-aware hard negative mining” are used to make MM-Embed work. This new method gets rid of the usual errors found in modality-specific language models. This lets MM-Embed correctly understand and get results from different data sources. MM-Embed has been fine-tuned across 16 different retrieval tasks using multimodal large language models (MLLMs). It is made to handle difficult real-world situations, such as zero-shot reranking for complex image-text searches.
Not only does MM-Embed do well on multimodal tasks, but it also does very well on text-only benchmarks. For example, it was in the top five on the MTEB test. It did very well in tests, with an average recall accuracy of 52.7% across all M-BEIR tasks. In particular, it showed a retrieval accuracy of 73.8% on the MSCOCO dataset, which shows that it can understand complex picture captions. MM-Embed improved ranking accuracy by over 7 points for composed image retrieval tasks like those in the CIRCO dataset. This was achieved through advanced LLM prompting for reranking.
Such success not only sets new standards for MM AI retrieval but also gives the directions for further developments in the AI systems that will be able to search for the relevant information in many types of formats that are linked to each other. In this regard, NVIDIA remains in the front line of utilizing artificial intelligence to its benefit in the form of MM-Embed which enhances search as well as eases information access across different formats.