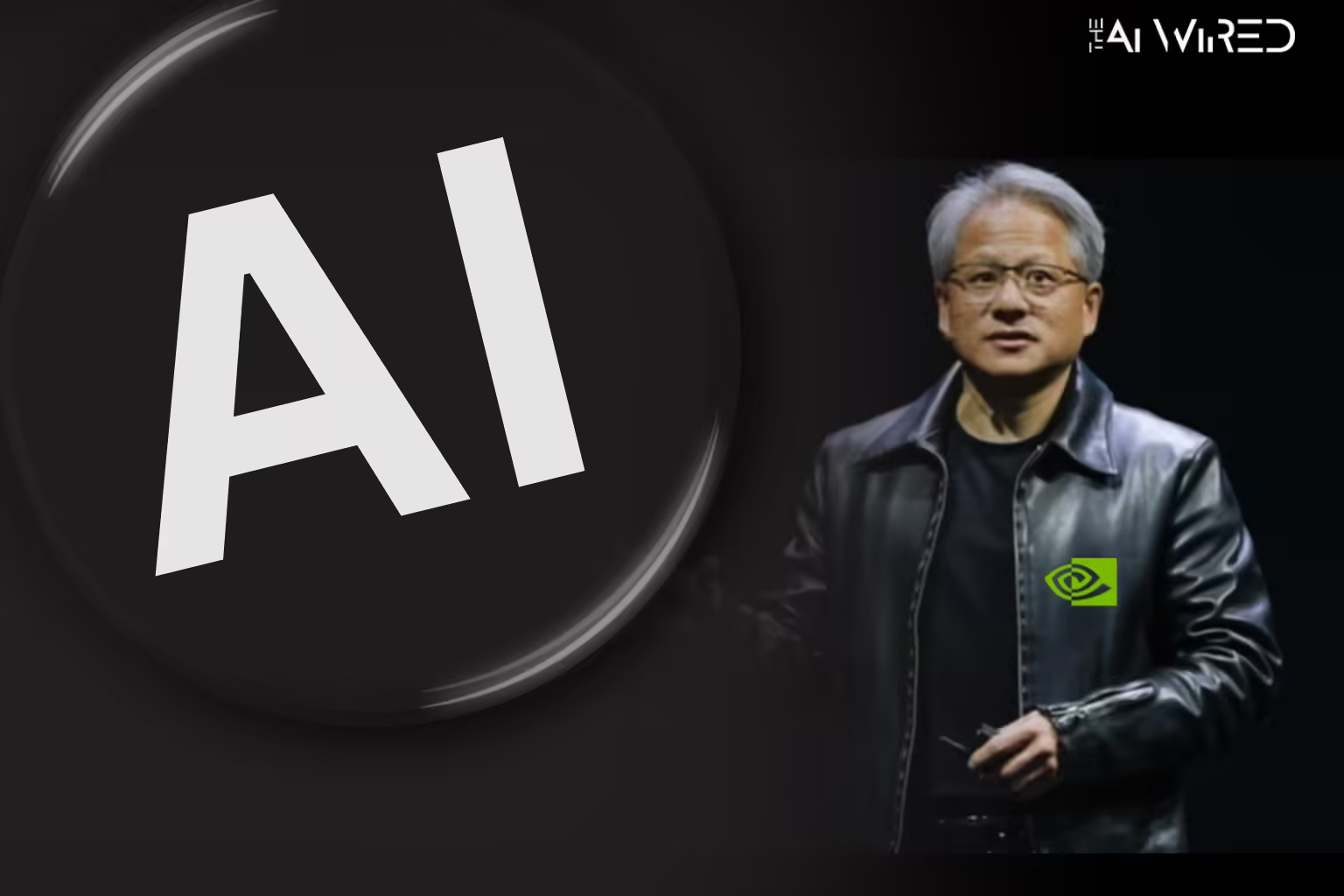
Nvidia Faces AI Scaling Challenges: The Shifting Paradigm of Growth
Nvidia’s dominance in AI hardware is under scrutiny as the industry questions the effectiveness of scaling laws. Discover how AI evolution impacts Nvidia’s future.
The meteoric rise of Nvidia as the global leader in AI hardware has been closely tied to the rapid scaling of artificial intelligence. However, cracks are appearing in the “scaling law” that has powered AI’s explosive growth—and Nvidia’s dominance.
AI Scaling Law: The Force Behind Nvidia’s Growth
The scaling law, which is an important part of current AI development, says that systems get smarter when they use a lot more data to build bigger models, which takes a lot of computing power. Companies like OpenAI, Google, and Anthropic have created a huge need for chip groups to train very large models, which has been made possible by Nvidia’s GPUs.
The launch of OpenAI’s ChatGPT marked a turning point in this method by showing how scaling could create AI systems that change the world. Morgan Stanley predicts that by 2025, Microsoft, Meta, Amazon, and Google will have spent more than $300 billion on capital projects. This year, they spent more than $200 billion.
Scaling Hits a Wall?
According to new information, scaling alone may not give as many benefits as it used to. Leaders in the field, such as Ilya Sutskever of OpenAI, now agree that the time of easy scaling may be coming to an end. Sutskever said, “The 2010s were the age of scaling, and now we’re back in the age of wonder and discovery.” This shows that people are becoming less sure that the old saying “bigger is better” still works when it comes to training AI models.
Researchers and executives in AI say that pre-training models have reached a plateau, which has made people turn their attention to post-training processes. CEO of Nvidia Jensen Huang says scaling is still important, but he also says it’s not enough by itself anymore. “Test time scaling” is a new area of research in which AI systems use more computing power to come up with better answers.
What This Means for Nvidia
Nvidia is in a very important spot right now. It has been so successful because the AI business can’t get enough chips, mostly for training very large models. But as people pay more attention to inference—that is, how models answer questions—demand for GPUs is expected to grow even more. Microsoft President Brad Smith says, “Right now, this is a market that’s going to need more chips, not less.” He says this because demand is expected to stay high for a long time.
Still, the long-term picture is still not clear. Businesses are still looking for the “killer app” for AI that will make these huge investments worth it. It’s important for Nvidia that Big Tech can get real results from AI deployments so they can get their money back for the billions they spent on infrastructure.
The scaling debate proves that AI’s future will not be defined by hardware only, although Nvidia remains dominant. While the industry attempts new methods, it adapts new concepts like AI models that can be deduced, and then Nvidia will need to evolve.