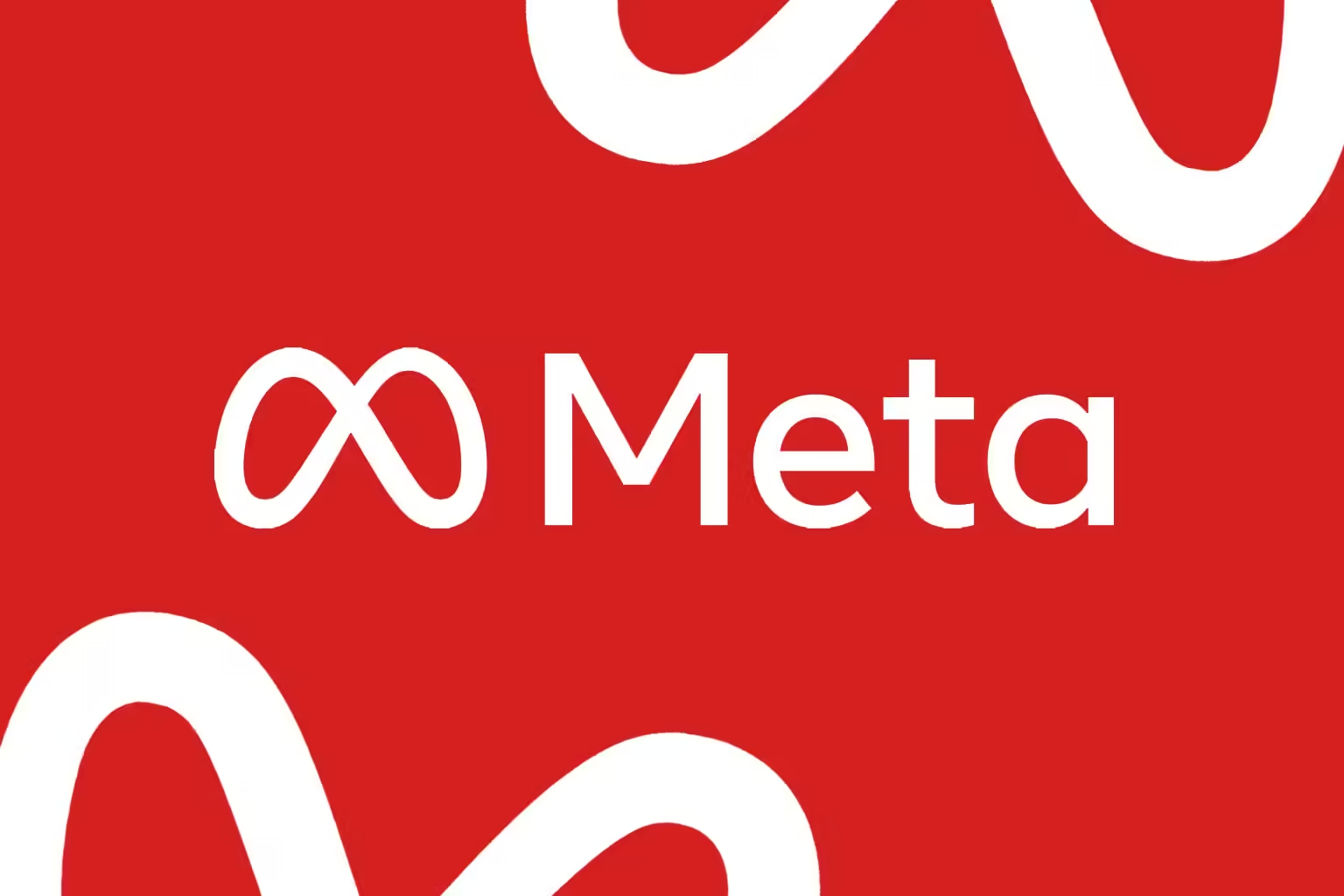
Meta AI Unveils Sparsh: A Breakthrough in Vision-Based Tactile Sensing for Robotics
Meta AI has launched Sparsh, the first general-purpose encoder for vision-based tactile sensing. This could lead to progress in robotics and AI by making touch-sensing solutions more flexible and effective.
This week, Meta AI released Sparsh, a cutting-edge general-purpose encoder designed to make vision-based tactile sense better. “Sparsh” is a Sanskrit word that means “touch.” The goal is to solve robotics problems that have been around for a long time by providing a flexible, scalable solution that works better than current models that are made for specific sensors.
A Breakthrough in Tactile Sensing Technology
In the past, vision-based touch sensors have had problems because they come in a lot of different designs and can’t be used in a lot of different robotic situations. Meta AI’s Sparsh tries to get around these problems by training on more than 460,000 unlabeled tactile images from a wide range of senses using self-supervised learning (SSL). With this method, Sparsh can skip the time-consuming and expensive process of collecting tagged data. This opens up new real-world uses in many areas.
Sparsh’s design includes advanced SSL models like DINO and Joint-Embedding Predictive design (JEPA), which lets it work with common tactile sensors like DIGIT and GelSight. Meta’s new idea includes TacBench, a test that measures six important things: estimating force, detecting slippage, estimating pose, determining grasp stability, recognizing textiles, and manipulating objects deftly. Sparsh always does better than traditional models. It can increase job success rates by up to 95% while only needing 33–50% of the labeled data that sensor-specific solutions need.
Sparsh’s release is significant for robotics, where being able to feel things is important for making robots engage and handle things like real people. Sparsh makes it easier for robots to connect with their surroundings. It can be used for everything from industrial automation to home robotics. The first tests show that Sparsh is very good at what it does. It did especially well on difficult jobs like detecting slips and fabrics, where it got the highest F1 scores of similar models.
The development of Sparsh by Meta AI is a promising step for embarking on the actual progression of physical intelligence. Sparsh helps eradicate the dependency on labeled datasets and provides effective channels for creating complex tactile apps applicable to dissimilar robotic platforms and territories. Despite this, relatively high performance in the TacBench benchmarks and its versatility indicate that similar fields that rely on complex tactile sensing could also benefit from the network.
Sparsh is becoming more well-known for its study of AI. Automation could move faster if it could be used for many things. This would make it easy to do things that need precise touch. It’s clear that Meta still wants to help AI experts and improve robots by giving them more power. Tactile sense technology that is more flexible and better at handling data would be great for robots.