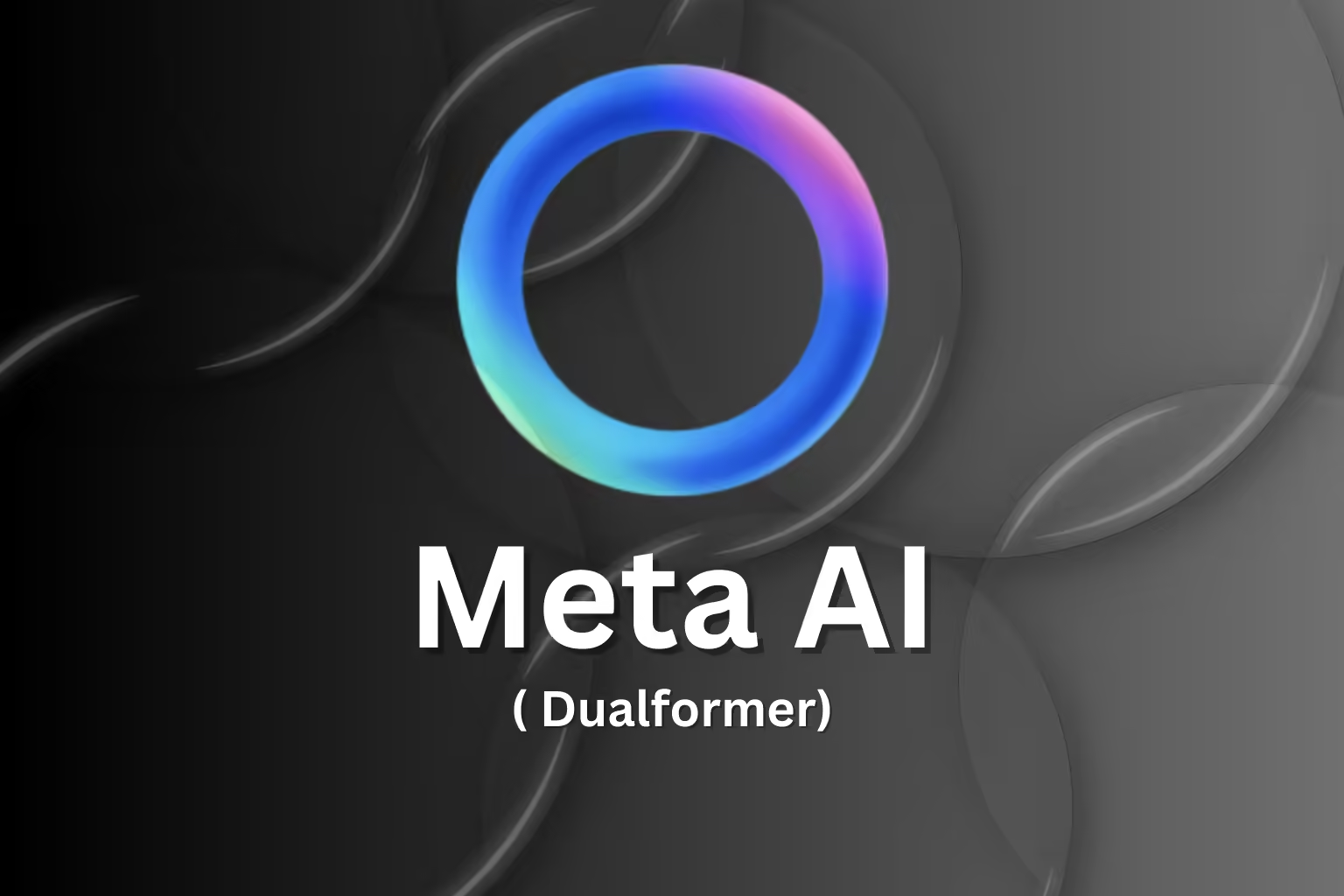
Meta AI Launches Dualformer: Revolutionary AI Model for Fast and Slow Reasoning
Meta AI’s Dualformer model uses both fast and slow thinking to improve the accuracy and efficiency of AI decision-making through adaptive cognitive modes.
Meta AI has unveiled Dualformer, a new type of model that expands on these characteristics and unifies the concept of how the mind works and rapidly makes near-instant decisions while at the same time also has a second layer that allows users to approach problems methodically.
Meta’s new Dualformer model claims to change how AI is thought about by combining two different ways of thinking: The two systems involve quick, problem-solving cognitive actions and decisions and slow, rational decision-making. This new feature allows the model to switch the way it operates depending on the difficulty of the work, making the work run faster and the decisions made more accurate. This is achieved by the Dualformer model through a unique combination of randomized reasoning traces and trace-dropping functions during the training process. This makes it different from other AI models which can only work at fast or slow thinking ability.
In the past, AI thinking models had to choose between being fast and accurate. While faster models, like solution-only systems, are designed to get results quickly at the cost of accuracy, slower models, like the Searchformer, offer high accuracy at the cost of time and computing power. When these modes are tried to be combined, they often need to be fine-tuned and controlled in new ways, which can make the system less flexible. The design of Dualformer eliminates these problems by letting the model switch between thinking modes without any help from outside sources. This makes the architecture simpler while making it more flexible.
Technical Innovations in Dualformer’s Architecture
Dualformer uses a transformer-based encoder-decoder architecture and a “trace-dropping” approach that acts like how people make decisions quickly. During training, methods like the A* search algorithm that create extraneous reasoning steps are taken out one by one. This way makes things run more smoothly by getting the model to generalize well while doing hard reasoning tasks.
Performance tests show that Dualformer is very accurate and uses computers very efficiently. It got an ideal answer rate of 97.6% on maze tasks in detailed (slow) mode, cutting the number of steps needed for reasoning by 45.5% compared to the Searchformer model. Dualformer got 80% optimality in its fast mode, which was much higher than the Solution-Only model’s 30%. In adaptive auto-mode, it kept a high optimal rate of 96.6% with almost 60% fewer steps than competing models. This balance shows how flexible Dualformer is by letting it switch between processing speeds based on the difficulty of the job.
The Meta AI Dualformer allows the usage of the high end of AI in many areas where fast and slow thinking has to be integrated into the best working fashion. The flexible decision-making approach reveals a new area for the application of AI in complex real-world problems.